REVEALED: How a Retail Giant Cut Security Costs 50% While Boosting Threat Detection
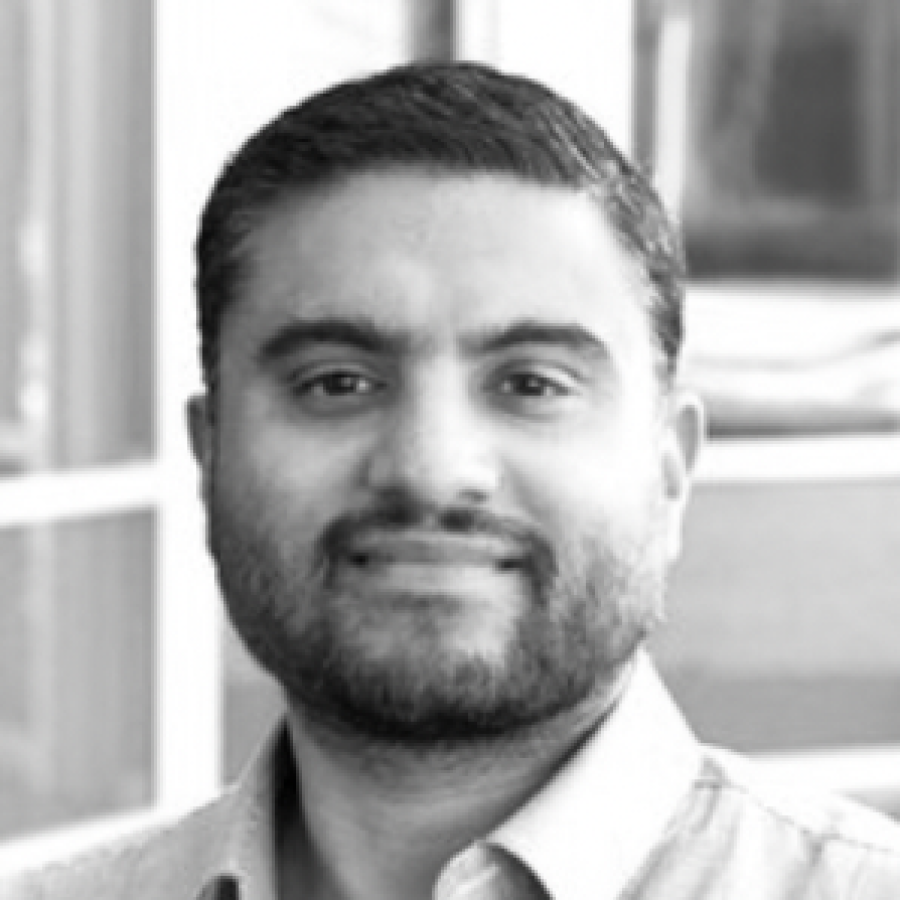
This is the third and final post in our "Data Intelligence in Security: The AI Pipeline Revolution" series. In Part 1, we explored why AI-powered security data pipelines have become essential for modern SOCs. Part 2 covered the critical capabilities to evaluate when selecting a solution. Today, we'll share implementation best practices and examine the business impact you can expect.
Implementing an AI-powered data pipeline represents a strategic investment that delivers substantial business value beyond simple cost reduction. When executed properly, these solutions transform security operations by enhancing visibility, accelerating detection, and freeing analysts from data management drudgery.
But even the most advanced AI technology will fail to deliver its full potential without proper implementation strategy. Let's explore the best practices that lead to success and the business impact you can expect.
Implementation Best Practices
Take a Phased Approach
Implementing your AI-powered data pipeline in stages dramatically reduces risk while accelerating time-to-value. This incremental approach builds confidence, secures stakeholder support, and allows your team to develop expertise through hands-on experience.
Consider this structured progression:
- Start with high-volume, low-complexity sources like firewall logs or VPC flow data that offer immediate cost benefits
- Implement parallel processing during initial phases to maintain continuous security visibility
- Conduct comprehensive validation comparing pipeline outputs with original data to verify security context is preserved
- Gradually incorporate additional data sources prioritizing those with the greatest ROI potential
- Continuously refine and optimize based on operational experience
One CISO who successfully implemented this approach noted: "We initially tried to migrate everything at once and struggled. When we switched to a phased approach starting with our highest-volume data, we saw immediate cost savings that funded the rest of the implementation."
Establish Clear Success Metrics
Well-defined metrics help maintain stakeholder support by demonstrating tangible benefits while guiding ongoing optimization. They also create accountability for both the implementation team and solution provider.
Track these key performance indicators:
- Data reduction percentage compared to baseline volumes
- Actual cost savings across SIEM licensing, infrastructure, and operations
- Performance impacts on query response times and alert processing
- Security effectiveness improvements in detection accuracy and false positive rates
- Analyst efficiency gains measured through time allocation studies
Establish these metrics during planning, measure before implementation for accurate baselines, and track consistently throughout deployment. A security operations leader shared: "Defining clear success metrics upfront was critical. When we showed a 63% reduction in data volume with zero impact on detection capabilities, we gained immediate support for expanding the implementation."
Prioritize Change Management
The most technically perfect implementation will fail if security analysts don't understand how to leverage its capabilities. A comprehensive change management approach addresses the human elements, ensuring adoption and maximizing value.
Effective change management includes:
- Early stakeholder engagement with security teams, IT operations, and compliance
- Role-specific training tailored to different team members' needs
- Process updates that incorporate the pipeline's capabilities into daily workflows
- Clear communication about implementation timelines and expected changes
- Feedback mechanisms for identifying issues and suggesting improvements
Organizations that neglect this aspect often struggle despite technical success. One security director observed: "The technology worked perfectly, but we initially failed to help our analysts understand how it changed their workflows. Once we addressed the human side with targeted training, adoption accelerated dramatically."
Implement Strong Data Governance
Establishing or enhancing data governance practices ensures appropriate management of security information throughout its lifecycle. Strong governance frameworks define how data should be collected, processed, stored, accessed, and eventually disposed of.
Comprehensive governance typically addresses:
- Data classification schemes based on sensitivity and security value
- Retention policies aligned with both compliance obligations and analytical needs
- Access control frameworks determining who can view different data types
- Quality standards for ensuring data accuracy and completeness
- Lifecycle management processes for data creation, transformation, and deletion
One compliance officer noted: "Implementing our security telemetry pipeline gave us the opportunity to completely revamp our security data governance. We now have consistent policies across all our security data, which has simplified compliance and improved our overall security posture."
Treat Vendors as Strategic Partners
The relationship with your solution provider significantly impacts implementation success and long-term value. The most successful implementations treat vendors as strategic partners rather than just technology providers.
Effective vendor relationships include:
- Clear definition of roles and responsibilities between internal teams and vendor resources
- Regular executive engagement beyond technical implementation contacts
- Structured knowledge transfer to build internal expertise
- Feedback loops that provide input for product roadmap enhancements
- Success reviews that objectively assess outcomes against expectations
Organizations that establish strategic partnerships typically report more successful implementations and greater long-term satisfaction with their chosen solutions.
Business Impact and ROI
Implementing an AI-powered security telemetry pipeline delivers substantial value across multiple dimensions. Understanding these benefits helps security leaders build compelling business cases and set realistic expectations.
Cost Reduction
The most immediate and quantifiable ROI comes from significant cost savings across multiple aspects of security operations:
- 40-60% reduction in SIEM licensing costs by limiting ingestion to high-value data
- 50-70% decrease in storage requirements through intelligent optimization
- 30-50% lower computational costs for security analytics
- 25-40% reduction in network transfer costs, particularly valuable in cloud environments
Case Study: How a Major Retailer Transformed Their Security Operations
A large American retailer provides an excellent example of the transformative impact AI-powered security data pipelines can deliver. Their journey highlights both the challenges many organizations face and the concrete benefits of implementing the right solution.
The Challenge
The retailer struggled with exploding costs related to their Splunk implementation as security data volumes grew rapidly. VPC Flow logs alone comprised nearly half of all data being ingested into Splunk, creating both financial pressure and operational challenges:
- Storage, egress, and compute costs were increasing at an unsustainable rate
- Query times were slowing significantly due to the massive data influx
- Manual efforts to randomly sample data created dangerous blind spots
- Daily ingest volume spikes frequently threatened to exceed limits
As the Sr. Director of Cyber Security Engineering described it: "Before Observo, we spent a lot of our time manually trying to control our security data volumes."
The Solution
After evaluating various options, the company implemented an AI-powered security telemetry pipeline that delivered:
- Intelligent Data Optimization: Using algorithms specific to each data type, they reduced log volume by more than 80% by eliminating low-value data and summarizing similar logs.
- Data Lake Creation: They established a full-fidelity data lake in AWS S3 where they sent all raw data in highly compressible Parquet format, costing just 1-3% compared to SIEM storage.
- Smart Routing: The solution enabled them to maintain a leaner Splunk index by dropping older data after initial analysis while retaining the ability to rehydrate data on demand.
- Anomaly Detection: By surfacing anomalies in the telemetry stream before indexing, they enriched security events with sentiment analysis for better prioritization.
The Results
The impact was transformative across multiple dimensions:
- 50% reduction in total infrastructure spend including storage and compute costs
- Expanded security visibility by adding new data types without stressing daily ingest limits
- Faster query performance through a leaner index and optimized data
- Simplified compliance processes with easy data retrieval using natural language queries
- Accelerated incident response by prioritizing critical events and reducing alert noise
"With Observo, we significantly cut infrastructure costs and added new data types for more efficient security," noted the Sr. Director of Cyber Security Engineering.
This real-world example demonstrates how the best practices and capabilities we've discussed throughout this series translate into concrete business value when properly implemented.
This cost efficiency creates financial flexibility to expand security visibility to previously unmonitored systems or invest in additional security capabilities without increasing overall budgets.
Security Effectiveness
Beyond cost savings, AI-powered pipelines significantly enhance security outcomes by improving detection accuracy, accelerating response, and enabling more comprehensive visibility.
Security teams implementing these solutions consistently report:
- 40% faster detection and response times through early anomaly identification
- 30-50% reduction in alert volume with corresponding increase in alert quality
- 25-35% improvement in detection accuracy for both known and novel threats
- 40-60% expansion in security visibility by analyzing previously excluded data sources
These improvements directly translate to reduced risk exposure by shortening the window between compromise and detection, enabling more rapid containment of threats before they cause significant damage.
Team Productivity
Security teams experience transformative productivity improvements when freed from data management drudgery and supported by enriched, optimized security information.
Organizations implementing AI-powered pipelines typically realize:
- 30-40% increase in analyst productivity through reduced data wrangling
- 25-30% shift from data management to proactive security activities like threat hunting
- 35-45% faster onboarding for new analysts due to more intuitive, context-rich data
- Improved job satisfaction and retention from reduced alert fatigue
This productivity enhancement allows security teams to handle greater alert volumes and more complex investigations without proportional staffing increases—a critical advantage given the ongoing shortage of skilled security personnel.
Compliance Efficiency
Meeting constantly evolving regulatory requirements represents a significant challenge for security teams. AI-powered pipelines streamline compliance processes by automatically collecting, formatting, and retaining required information.
Organizations leveraging these solutions for compliance typically achieve:
- 30-50% reduction in compliance reporting effort through automated data collection
- 40-60% decrease in privacy-related incidents through comprehensive PII detection
- 70-90% cost reduction for long-term compliance data storage via optimized formats
- Faster, more thorough regulatory audits with readily available evidence
A financial services organization demonstrated these benefits when they found they could maintain seven years of security data for regulatory purposes at less than 10% of their previous cost.
Business Agility
Perhaps the most strategic benefit is the increased business agility enabled by removing data constraints from security operations. When security teams can rapidly incorporate new data sources and adapt to changing environments without proportional cost increases, security becomes an enabler rather than a constraint on innovation.
Forward-thinking organizations leverage this agility to achieve:
- 30-40% faster security enablement for new business initiatives through streamlined data onboarding
- 50-70% reduction in security-related delays for cloud migration and digital transformation
- Enhanced security support for acquisitions and divestitures through flexible data integration
- More collaborative relationships between security and business teams based on responsive support
This improved agility directly impacts business outcomes by reducing time-to-market for new offerings and enabling more responsive adaptation to changing market conditions—all while maintaining or enhancing security posture.
Future Trends: Where Security Data Management Is Heading
As you implement your AI-powered security telemetry pipeline, it's worth considering emerging trends that will shape future security operations:
Agentic AI
Agentic AI represents a revolutionary advancement beyond traditional machine learning in security operations. These systems combine multiple AI models with autonomous decision-making capabilities to solve complex security challenges with minimal human intervention.
We're already seeing early implementations with:
- Autonomous investigation workflows that follow security playbooks without human direction
- Dynamic tool orchestration where AI agents select specialized tools as needed
- Self-directed enrichment that identifies information gaps and seeks additional context
- Adaptive response planning that generates and implements remediation strategies
One CISO noted: "We've seen our first agentic AI security systems reduce investigation time by 70% for common incidents. The system doesn't just flag anomalies—it autonomously follows our investigation playbook, gathers context, and presents a comprehensive assessment."
Edge Processing and Distributed Intelligence
As data volumes grow and latency requirements become more stringent, we're seeing a shift toward processing security telemetry closer to its source through distributed intelligence at the edge:
- Local preprocessing and filtering before central transmission
- Edge-based detection capabilities that identify threats without requiring central analysis
- Federated learning models that improve without centralizing sensitive data
- Intelligent agents deployed throughout the environment
This approach is particularly valuable for remote locations, cloud environments, and IoT deployments where centralized collection creates bandwidth and latency challenges.
Privacy-Preserving Analytics
As privacy regulations expand globally, we're seeing growing emphasis on technologies that enable security analysis without exposing protected data:
- Homomorphic encryption enabling analysis of encrypted data without decryption
- Secure multi-party computation allowing collaboration without revealing underlying data
- Differential privacy techniques that extract insights while protecting individual identities
- Zero-knowledge proofs to verify security conditions without revealing sensitive information
These techniques will become increasingly important as organizations balance comprehensive security visibility with stringent privacy protection.
Your Action Plan: Next Steps
As you consider implementing an AI-powered security telemetry pipeline, these practical next steps will help you move forward:
- Assess your current state by measuring data volumes, costs, and operational metrics
- Define clear objectives aligned with business priorities
- Evaluate potential solutions through hands-on testing with your actual data
- Develop a phased implementation roadmap with clear success metrics
- Create a comprehensive change management plan to ensure adoption
- Establish governance frameworks for long-term sustainability
The security data explosion shows no signs of slowing. If anything, it will accelerate as organizations continue to digitize operations and expand cloud adoption. Traditional approaches that simply scale up resources to accommodate this growth are financially unsustainable and operationally inefficient.
AI-powered security data pipelines aren't merely cost-cutting tools—they represent a fundamental shift in how organizations approach security data management. By intelligently processing security data before it reaches expensive analytics platforms, these solutions enable security teams to achieve what previously seemed impossible: comprehensive visibility at sustainable cost.
The most successful security leaders recognize that effective security isn't just about having more data—it's about having the right data, properly enriched, delivered to the right tools, at the right time. AI-powered pipelines make this vision achievable, transforming security operations from a constant struggle against data volume into a strategic capability that provides genuine business value.
If you found this interesting, take the next step with our CISO Field Guide to AI Security Data Pipelines—a deeper dive into expert insights, real-world use cases, and strategies for transforming your security data operations.