Observability 101: The Evolution of Observability: From Log Management to AI-Driven Analytics
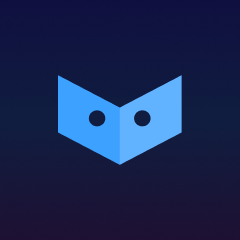
Introduction
In today's fast-paced, digital-first world, the seamless operation of software systems and applications is critical for businesses and organizations across industries. The ability to gain deep insights into the inner workings of these complex digital ecosystems has led to the rise of observability as a foundational practice. Observability, in essence, empowers organizations to understand the health, performance, and behavior of their applications and infrastructure in real-time. It's the compass that guides IT teams through the maze of modern technology, enabling them to troubleshoot, optimize, and ensure reliability at scale.
The fascinating journey of observability has been nothing short of a technological odyssey, evolving from its rudimentary origins as basic log management to the highly sophisticated AI-driven analytics systems we have today. This transformation has been driven by a relentless pursuit of better ways to understand, monitor, and manage the increasingly intricate digital landscapes upon which our daily lives and business operations depend.
In this comprehensive exploration of the evolution of telemetry and observability, we'll embark on a voyage through time, tracing the remarkable trajectory from its modest beginnings to the current state of the art. Along this journey, we'll delve into key milestones, breakthrough technologies, and paradigm shifts that have reshaped the observability landscape. From the days when logs were primarily viewed as troubleshooting aids to the dawn of predictive and proactive observability driven by artificial intelligence, we'll uncover the pivotal moments that have defined this field.
So, fasten your seatbelts and prepare to dive deep into the heart of observability's evolution, where data-driven insights and intelligent automation are shaping the way we perceive, manage, and optimize the complex web of interconnected systems that drive the digital world. Join us on this captivating expedition as we unravel the past, present, and future of observability and its profound impact on the way we engineer, monitor, and sustain the digital infrastructures of tomorrow.
Log Management: The Foundation
The journey of observability begins with log management. Logs are records of events, activities, and actions within a system or application. Initially, logs were primarily used for debugging and troubleshooting purposes. System administrators and developers relied on logs to identify issues and diagnose problems. Log management tools allowed them to collect, store, and search through log data efficiently.
Key milestones in log management include the introduction of structured logging formats and the development of log aggregation tools like syslog and Splunk. These advancements made it easier to analyze and extract meaningful information from logs, but they were still reactive in nature, requiring human intervention to identify and address issues.
Metrics and APM: Expanding the Horizon
As technology evolved, so did the need for more comprehensive observability solutions. The introduction of metrics and Application Performance Monitoring (APM) tools marked the next step in this evolution. Metrics provide a more structured way to collect data about system and application performance, while APM tools focus on monitoring the end-user experience and transaction traces.
These advancements allowed organizations to proactively monitor their systems and applications, enabling faster response times to performance degradation and other issues. Tools like Prometheus, Grafana, New Relic, and Datadog became essential for DevOps teams and system administrators.
Tracing and Distributed Systems: A Complex Challenge
With the rise of microservices and distributed systems, observability faced new challenges. Understanding the flow of requests and transactions across a complex network of services became crucial. Distributed tracing emerged as a solution to this problem, providing end-to-end visibility into how requests traverse through various components.
Projects like Zipkin, Jaeger, and OpenTelemetry have played a significant role in advancing distributed tracing. These tools help organizations gain insights into latency, bottlenecks, and dependencies between services, improving the overall performance and reliability of their applications.
The AI Revolution: Predictive and Proactive Observability
The most significant leap in the evolution of observability comes with the integration of artificial intelligence (AI) and machine learning (ML) techniques. AI-driven observability solutions leverage these technologies to make sense of vast amounts of data generated by modern applications and systems.
a. Anomaly Detection: AI models can identify abnormal behavior within logs, metrics, and traces, helping organizations detect issues before they impact users. These models learn from historical data, making them more effective at identifying patterns that are indicative of potential problems.
b. Root Cause Analysis: AI-driven analytics can automatically pinpoint the root cause of issues by analyzing the relationships between different observability data points. This reduces the mean time to resolution (MTTR) and minimizes manual investigation efforts.
c. Predictive Maintenance: AI can predict when hardware or software components are likely to fail based on historical data and usage patterns. This allows organizations to proactively address issues and avoid costly downtime.
d. Auto-Scaling: AI-driven systems can also optimize resource allocation by automatically adjusting the number of instances in a cluster based on real-time traffic and performance metrics, ensuring optimal cost-efficiency.
e. Log and Event Correlation: AI models can correlate logs, events, and alerts from various sources, helping organizations gain a more holistic view of their systems and applications.
Conclusion
Observability has come a long way from its humble beginnings in log management. It has evolved into a sophisticated discipline that combines metrics, tracing, and AI-driven analytics to provide a comprehensive understanding of modern applications and systems.
As organizations continue to adopt complex, distributed architectures and deal with ever-increasing data volumes, AI-driven observability will become increasingly crucial. It empowers teams to detect and address issues before they impact users, optimize system performance, and make data-driven decisions to enhance reliability and customer satisfaction. An AI-powered observability pipeline like Observo.ai can help control costs, increase flexibility, and make your security and DevOps teams more productive.
The evolution of observability is ongoing, and the future promises even more exciting developments as AI and ML continue to advance. As technology continues to evolve, so will the tools and techniques we use to gain insights into the systems and applications that power our digital world.