Beyond Cost Cutting: The Hidden Benefits of Optimized Security Data
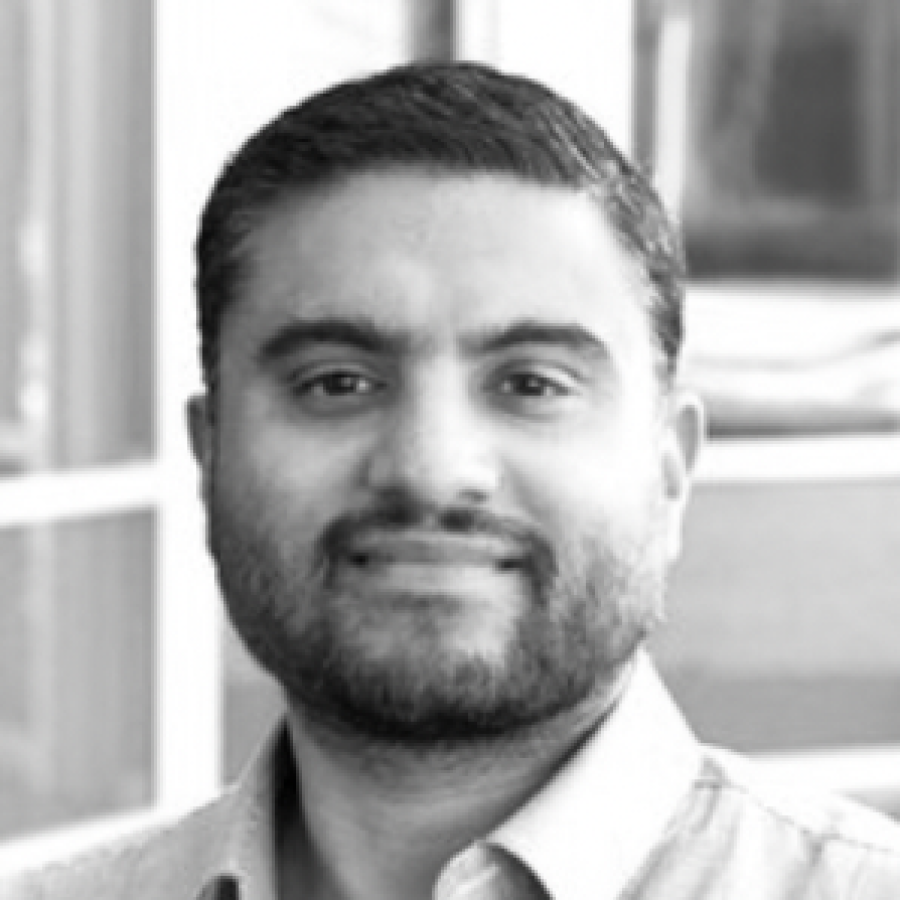
For many organizations, the first motivation to modernize their security data infrastructure is cost. And understandably so—data volumes are exploding, and the costs of storing and analyzing everything in a traditional SIEM can quickly become unsustainable.
But in my experience, cost savings are just the entry point. The true value of optimizing security data goes much deeper.
When you reduce noise, enrich your telemetry with meaningful context, and route data intelligently across tools and teams, you're not just saving money—you’re enabling better decisions, faster investigations, and more resilient operations. You move from reactive firefighting to proactive defense.
Optimization isn’t just a way to reduce costs. It’s a practical strategy for helping security and engineering teams work faster, see more clearly, and stay focused—especially when time and attention are limited.
Here’s what that shift looks like in practice.
Less Noise = Faster Detection and Resolution
Security teams today are drowning in telemetry. Somewhere in that flood of logs and events are the signals that actually matter—but first, someone has to find them.
The more strategically mature teams are the ones taking steps to reduce noise at the source. By identifying, filtering, and aggregating redundant or low-value events early in the process, they can focus their attention on high-signal data. The impact is immediate: fewer distractions, fewer false positives, and a much faster path to meaningful investigation.
It’s an analogy we’ve used before, but it holds true—especially in high-stakes environments: finding critical insights in your data is like searching for a needle in a haystack. Shrink the haystack, and you not only find the needle faster, you make the entire search less exhausting. And when that needle is a lateral movement or a privilege escalation, speed isn’t just a metric—it’s the difference between containment and compromise. Optimized data shortens the time between suspicious activity and decisive action.
Less Alert Fatigue, More Analyst Focus
One of the most overlooked sources of operational drag in security teams is alert fatigue. When every event is treated with equal urgency, analysts end up reacting to noise instead of responding to real threats. It’s a recipe for burnout—and worse, for missed signals that actually matter.
A more strategic approach involves prioritizing context-rich data earlier in the pipeline. By embedding enrichment and filtering closer to the point of collection, organizations can reduce the sheer volume of irrelevant alerts that reach analysts. The goal isn’t just fewer alerts—it’s better ones.
This shift allows teams to focus on high-confidence signals, make faster decisions, and preserve analyst capacity for higher-value work like threat hunting, investigation, and response. Alert fatigue isn’t just a morale issue—it’s a visibility issue. Reducing it should be a core part of any modern security strategy.
Enriched Data = Smarter Decisions
Reducing data volume is important—but it’s not enough. If the remaining data lacks context, you're just working with a smaller set of incomplete information. The teams that move fastest are the ones who invest in enrichment early in the pipeline—not as a downstream add-on.
That can include integrating threat intelligence to flag known indicators of compromise, applying GeoIP data to better understand where traffic originates, or tagging events with business system metadata to help teams quickly assess relevance and impact. These strategies turn raw events into something analysts can act on—not just collect.
Smarter data leads to smarter detection logic and faster investigations. Enrichment isn’t overhead—it’s what turns information into insight.
Smarter Alert Prioritization Starts at the Source
Not all anomalies are worth chasing. One of the most practical ways teams can scale their efforts is by distinguishing between what’s unusual and what’s actually important.
The most effective SOCs apply techniques like behavioral baselining and sentiment scoring to surface the anomalies that resemble known attack paths, or that show a higher likelihood of operational impact. When you’re staring at hundreds of log lines, a ranked list is better than an open-ended question.
Machine learning is especially good at comparing large volumes of data to find patterns—surfacing anomalies that might go unnoticed by even experienced analysts. But identifying something unusual isn’t enough. The real value comes from using those patterns to focus analysts on potentially critical incidents. When alerts are prioritized based on likelihood of impact—not just being different—teams can spend their time investigating the right signals. In a high-volume environment, that kind of focus can dramatically reduce response times and improve outcomes.
Compliance Is More than a checklist, It’s a Data Strategy
One of the more underappreciated risks in security telemetry is how often sensitive data shows up where you don’t expect it. Consider a routine support call to your insurance provider: you're asked to state your member ID, which happens to be your Social Security number, and the call is recorded "for accuracy and training purposes." That recording may be transcribed to text, passed through voice analytics, and eventually logged somewhere in your system.
Security and compliance leaders can’t rely on static rules to catch everything. They need systems that can recognize personally identifiable information (PII) in messy, unstructured, or unexpected formats—and take action.
More teams are moving toward dynamic data classification and inline masking—especially in pipelines—so they can detect and redact sensitive data before it’s stored, routed, or exposed to the wrong team. This approach doesn’t just protect your users—it simplifies audit readiness and lowers the risk of regulatory violations downstream.
Collect Once, Transform Accurately, Deliver Everywhere
Different teams use different tools—and that’s okay. What becomes a problem is duplicating data across those tools or forcing teams to work from a single platform just to reduce volume.
A more sustainable strategy is to design your pipeline to support intelligent, purpose-driven routing. That means sending enriched alerts to your SIEM, long-term data to a cost-effective archive, and metrics to observability tools—all from the same stream of telemetry.
This kind of flexibility reduces redundancy, lowers ingest costs, and ensures every team gets the data they need in the format they expect—without being forced to compromise with data blindspots or tool limitations.
Faster Queries with a Leaner Index
In many environments, the SIEM becomes a performance bottleneck—not because it’s underpowered, but because it’s overloaded. The more logs you push into it, the harder it becomes to run fast, meaningful searches.
One of the fastest ways to accelerate investigations is to optimize what goes into the index in the first place. If you reduce noise, transform schema, and enrich upstream, the data that lands in your SIEM is more relevant, more usable—and lighter.
Smaller indexes mean faster queries, quicker dashboards, and less time wasted waiting for results. In incident response, seconds matter. Making the dataset leaner is often the simplest way to make the system smarter.
Cloud-Native Data Lakes for Faster Breach Investigations
Data lakes are often framed as a cost-saving tactic—and that’s not wrong. Storing security telemetry in cloud-native infrastructure is dramatically cheaper than keeping everything live in a SIEM. But cost is only part of the story.
The real value of a well-structured data lake shows up when a breach investigation begins. Most compromises aren’t discovered the day they happen. They’re often traced back to events that occurred months earlier. At that moment, you don’t want to rely on cold storage, third-party retrievals, or manual reindexing just to start asking the right questions.
The better approach is to design your architecture so that historical telemetry remains queryable on demand—structured, searchable, and available for rehydration when needed. Whether it’s natural language search, partial data reprocessing, or streaming specific events back to your analytics tools, accessibility matters just as much as retention.
This isn’t just about compliance or forensics—it’s about ensuring you can reconstruct what happened, act with confidence, and prevent the same scenario from playing out again. A modern data lake isn’t just cheap storage. It’s a critical part of your incident response strategy.
Turning Security Data Into Strategic Advantage
Security teams face are challenged with more data than ever, but less clarity about what truly matters. The answer isn’t simply to collect more—it’s to make what you collect more useful. That means reducing noise, enriching signals, routing data purposefully, and retaining what’s necessary without dragging down performance or cost.
When these strategies come together, the impact goes beyond budgets. Teams respond faster. Investigations go deeper. Compliance becomes easier. And perhaps most important, analysts regain time and focus to do the work that actually secures the organization.
Getting there isn’t about ripping out existing tools—it’s about rethinking how your data moves, how it's transformed, and who it's really serving. Because when your telemetry is working for you—not against you—security becomes not just a function, but a capability that helps the business move faster, with confidence.
If you’re rethinking how your security data is managed, we’d be glad to share what we’re seeing across the industry—and how Observo AI is helping teams reduce noise, improve clarity, and respond faster. Reach out to schedule a demo and learn more.
To explore the ideas in this piece further, download our CISO Field Guide to AI Security Data Pipelines for real-world examples, key design principles, and practical strategies you can apply in your own environment.